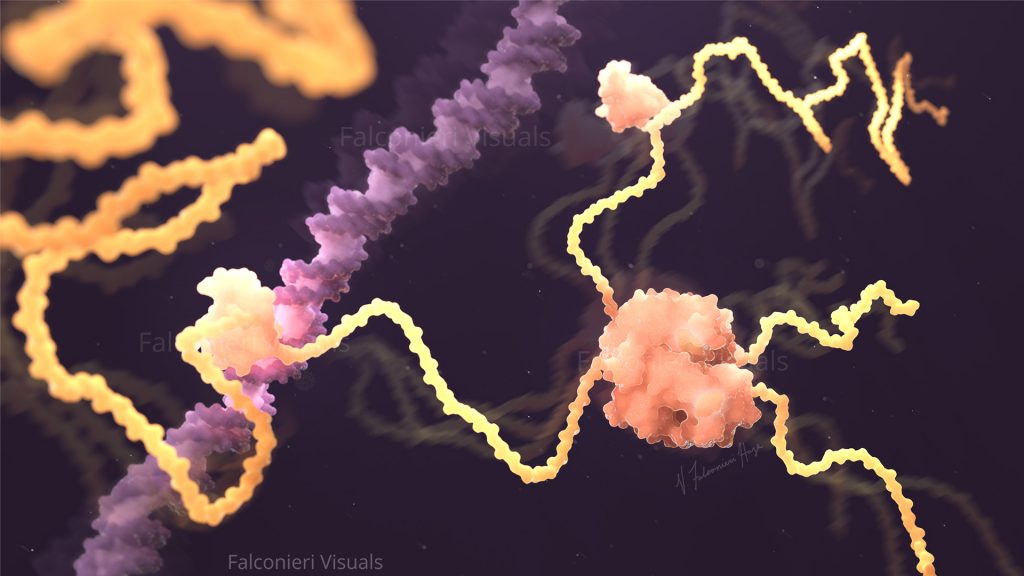
What is AlphaFold?
Predicting protein structures based on their amino acid sequence has been a scientific dream for decades. AlphaFold, an artificial intelligence (AI) system developed by DeepMind, makes that dream a reality. It predicts a protein’s 3D structure based solely on its primary structure.
This video from one of DeepMind’s research scientists, Jonas Adler, shows visually how AlphaFold works to iteratively predict and refine a structure.
Some of the graphics are really cool too. Here’s an illustration of how #AlphaFold iteratively builds a protein over almost 200 iterations. pic.twitter.com/01BPFFR8kZ
— Jonas Adler (@JonasAAdler) July 15, 2021
AlphaFold’s predictions are now available in the AlphaFold Protein Structure Database. The database includes 98.5% of the proteins in the human proteome, in addition to predictions for several other key organisms’ proteomes.
In comparison, the experimentally derived models deposited in the Protein Data Bank (PDB) to date only cover 35% of human proteins. Many of the PDB models are only fragments of entire proteins, whereas AlphaFold provides a full-length prediction for every protein. (Which is amazing. Absolutely astounding!)
However, not every structure is predicted with high confidence. More on that below.
How can medical illustrators use AlphaFold?
When building a molecular model for an illustration or animation, AlphaFold’s predicted structures can help fill in the gaps between experimentally determined structures.
Structures in the PDB come primarily from X-ray crystallography, cryo-electron microscopy, or NMR experiments. Each experiment requires a considerable investment of time and resources to produce high quality data, and even then some proteins have yet been too intractable to resolve. As a result, there are many proteins that have either no or only partial structures deposited. Before AlphaFold, the only alternatives were to represent the protein schematically or to try and find a related protein that might look similar.
However, in these cases, medical illustrators can now look for a predicted structure in AlphaFold’s database, and if the predicted structure is reasonable (see Limitations of Predictions, Evaluating a Predicted Structure, below), that structure can be confidently used in a molecular visualization as a reasonable hypothetical stand-in when no experimental structure exists.
Limitations of Predictions
Now, before you get too excited (trust me, I got too excited), there are some limitations to keep in mind.
Flexible regions
AlphaFold can only guess at conformations of very flexible regions. These regions might be intrinsically disordered, and are bobbling about at random in reality, or they may only form a structure when in complex with another protein. (AlphaFold’s predictions currently only account for one protein at a time).
Within an AlphaFold entry’s 3D view, you can see these low confidence regions highlighted in yellow and orange (EGFR shown below).
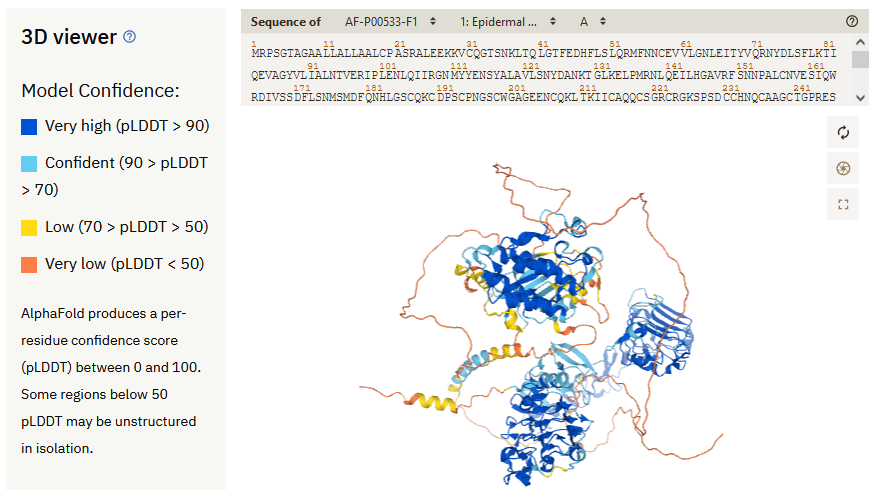
The researchers behind AlphaFold recommend that these regions “should not be interpreted as structures, but rather as a prediction of disorder”.
Proteins with rigid domains connected by flexible linkers also pose a challenge for AlphaFold. AlphaFold will guess at the conformation for that linker, and the confidence for the resulting orientation of the two domains relative to each other will be low. However, in the 3D view, both regions might be colored entirely blue, indicating high confidence. This misses the nuance of the low confidence of the domain orientations. The best place to identify this issue is the Predicted Aligned Error plot in each entry. How to read these is very helpfully explained right below the plot in the database. I’ve also included an example Predicted Aligned Error plot for a protein (EGFR) with a few rigid confident domains connected by flexible linkers with an annotated interpretation.

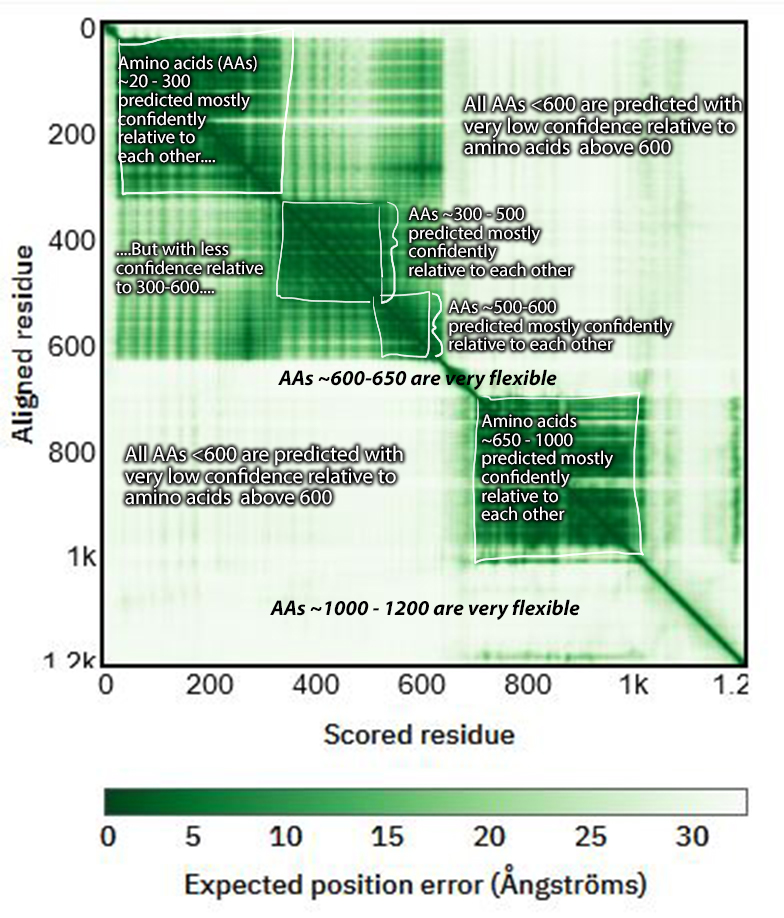
Issues with some membrane proteins
The EGFR example also brings up another limitation – AlphaFold doesn’t factor in the separation of domains by membranes. As a receptor tyrosine kinase (RTK), EGFR has an extracellular region, a transmembrane region, and an intracellular region. However, AlphaFold’s prediction is all crumpled up on itself with the intracellular domain interacting with the extracellular domain. Obviously these domains would be separated by the plasma membrane in a cell.
However, some membrane proteins can still be predicted quite confidently – see TRPV1 and GPCRs like rhodopsin.
Complexes
Some proteins form complexes with other proteins. Alphafold does not capture these complexes – it only shows one protein at a time. (So for a protein like hemoglobin, which consists of 2 alpha and 2 beta chains in a tetramer, AlphaFold only predicts the alpha chain and the beta chain conformations alone).
Another challenge of complexes is that interacting proteins may dramatically change the shape of the protein of interest. It is also possible for the other proteins to interact with and order disordered regions. Currently AlphaFold cannot predict either of these cases.
Multiple conformations
The #AlphaFold is an extraordinary accomplishement by @DeepMind @emblebi and @demishassabis. It’s a great tool ALONGSIDE #CryoEM structure determination and experiments.
— Alexey Amunts (@A_Amunts) July 23, 2021
Here is why: 2 conformations resolved by cryoEM, whereas AF2 produces an intermediate state. Just an example. pic.twitter.com/jLFtSLwSbz
In this example highlighted by Alexey Amunts, cryo-EM experiments have found 2 distinct conformations for a protein. However, AlphaFold only predicts an intermediate. The cryo-EM tells us that this protein flips back and forth between these conformations, whereas the AlphaFold prediction may lead one to hypothesize that there is one static conformation.
Evaluating a Predicted Structure
So with all this in mind, here are key points to review before using a predicted structure.
- Are there experimental structures available for the protein? How do they compare?
- How much of the protein is confidently predicted?
- For most molecular visuals, a pLDDT score above 70 will suffice. This indicates that at least the backbone, if not the side chain directions, is correct.
- Does the protein usually form a complex that might change its shape?
- Does the protein usually form a higher order multimer? (I.e. dimer, trimer, etc.)
- Are there multiple rigid domains connected by flexible linkers? If so, is the relative orientation of the rigid domains confidently predicted or are they aligned more or less at random? (See the predicted aligned error plot)
References
1. Jumper J, Evans R, Pritzel A, et al. Highly accurate protein structure prediction with AlphaFold. Nature. July 2021:1-7. doi:10.1038/s41586-021-03819-2
2. Tunyasuvunakool K, Adler J, Wu Z, et al. Highly accurate protein structure prediction for the human proteome. Nature. July 2021:1-9. doi:10.1038/s41586-021-03828-1
1 Comment
How Science and the Arts are Intermixing in the BioHealth Capital Region · BioBuzz · October 25, 2022 at 3:52 pm
[…] into the research, diving into literature and utilizing tools like the PDB Protein Data Bank and AlphaFold to understand all the nuances of how proteins and molecules move, bend, and interact with one […]
Comments are closed.